Data-driven decision making has become a necessity for the best businesses to stay on top of their daily operations and respond to areas for improvement. However, the quality of this data will greatly affect how useful it will be. Maintaining a high quality of data allows you to make decisions that improve customer relations and increase revenue. Poor quality data informs poor decisions and restricts access to customers while also using up time and resources to correct.
Because of its capabilities, data should play a vital role in informing your business decisions. As a result, your business should have a clear and well-thought-out plan for how data will be collected, maintained and analyzed. Improving your data quality through careful data collection and maintenance should be a key part of your business operations and will set your business apart from the others.
Sign up for a free trial!
Benefits of improved data quality
Improved data quality gives you a complete, accurate picture of your business and the customers you serve, allowing you to make more informed decisions that save time and money. High-quality data can be used to inform marketing campaigns that increase customer engagement, develop more trust in customer relationships and receive a higher return on investment in sales and marketing strategies.
Access to accurate data takes the guesswork out of key business decisions, increasing efficiency and saving costs. By following just a few steps, you can set your business up for success with a clear plan for collecting more valuable data and using it to inform critical business decisions.
Steps to improve data quality
According to our Data Quality Benchmark report, just 20 percent of organizations have a centralized data quality strategy under one owner. This means most companies see a lot of different, departmental and disconnected strategies, resulting in less effective data quality efforts.
By assigning a single data owner (including considering hiring for the relatively new title of chief data officer), you benefit from having one person taking the responsibility for the quality and standards around your data assets. In fact, this centralization even correlates with company profits: more companies who manage their data quality with one single owner have enjoyed a significant increase in profits in the last 12 months.
This central data owner should also be supported by a team of data professionals—data stewards, data service officers, analytics professionals, data scientists, etc.—who can help enforce policies and promote the use of data to drive insights across the business.
Implement proactive processes
Improving your data quality requires careful and constant consideration to help your business maintain a strong and effective data strategy. Your business should develop a clear plan for using and analyzing data as well as keeping that data up-to-date and accurate. To improve and sustain your business’s data quality, you should carry out the following steps:
1. Develop your objectives
Data quality means something different across different organizations. For some, it’s ensuring that customer contact data is accurate so that shipments are received in a timely manner. For others, it could be complete prospect profiles that help with marketing segmentation efforts. At its heart, data quality is about being fit for a desired purpose.
In order to collect meaningful data, your business should have clear objectives in place for how this data will be used to improve decision-making and daily operations. When developing your objectives, you should consider the following factors:
- Your business goals
- How you measure progress towards goals
- What data you need from customers and clients
- How this data will be used and analyzed
2. Assess your current data
Before implementing any data quality improvement plan, you need to understand where you stand today. Using the data quality sophistication curve, you can determine where you are related to data management efforts and what your next steps should be. There are four stages of sophistication your organization can fall into:
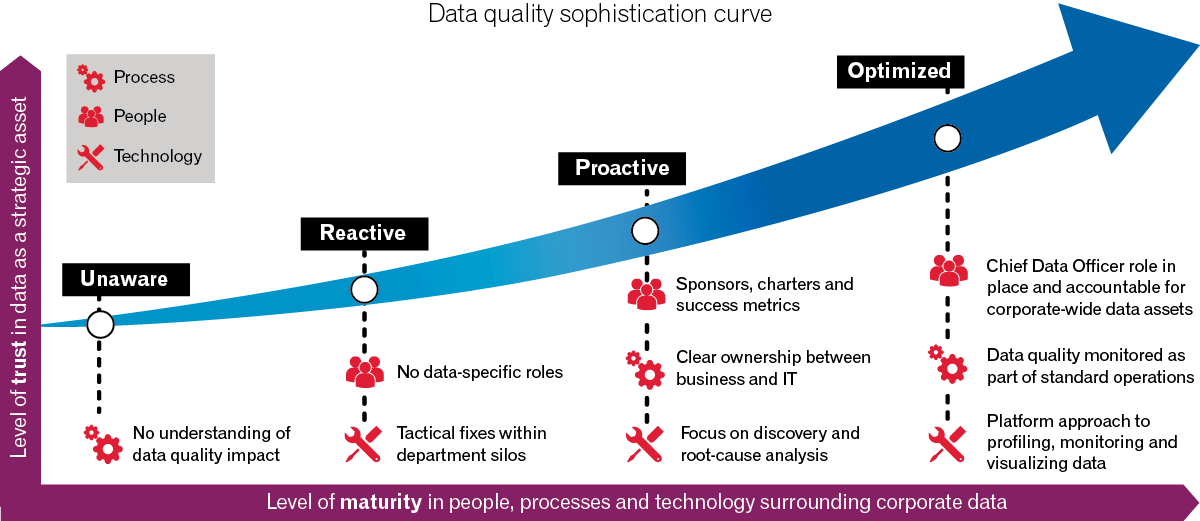
Almost half of organizations today fall into the reactive or unaware stage, meaning there is a lot of room for data quality improvement.
Data assessment should be a continuous process. The most successful businesses are constantly assessing the quality of their data and the effectiveness of their current data management plan. A constant reassessment of data allows a business to react to areas of concern and make improvements when necessary.
3. Cleanse your existing data
Inaccuracies or inconsistencies in your data will harm your data quality and prevent you from using it for effective decision-making. Over time, data sets become riddled with out-of-date or incorrect information as customer data changes or information is improperly added to your database.
Data cleansing is the process of correcting incomplete or inaccurate information, fixing formatting issues and more. Issues in your data set that require data cleansing include:
- Typos in data entries
- Incomplete data fields
- Inconsistent formatting of addresses or contact information
- Out-of-date contact information
- Duplicate data entries
Addressing incorrect information in your database provides you with more confidence that your data provides an accurate picture of your customers and allows you to make appropriate business decisions as a result. There are a variety of tools available to help you cleanse your data. These tools include email address list cleansing, real-time address verification and more to help you cleanse your database of errors and also prevent incorrect information from entering at all.
4. Establish a data collection and management plan
Because data collection issues are nearly inevitable, you should have a carefully considered plan in place to prevent these issues from arising and addressing them if they do. Being proactive with your data collection allows you to build a database of accurate and informative data instead of a database that needs to be fixed later. This will allow you to spend more time focusing on making decisions than fixing your data.
Considerations for an effective data collection and management plan include preventing poor quality data from entering your database and consistently assessing the quality of the data you already have. Methods to include in an effective plan include:
- Using tools like real-time email verification to ensure accuracy upon entry
- Creating required fields on forms to avoid incomplete information
- Regular, periodic data cleansing to catch errors or outdated information
- Providing appropriate training to all staff involved in data assessment and decision-making
Having a clear data collection and management plan will save your business valuable time and money correcting mistakes and allow you to be confident in using your data for strategic purposes.
5. Develop a plan for maintenance and improvement
In order to maintain strong data quality and business growth, your business should have a plan in place to regularly assess the quality of data and its use in decision-making. Data-driven decision-making is a constantly evolving strategy.
Effective plans for maintenance and improvement require consistent buy-in from everyone in the business. A data-driven business should foster a healthy culture of improvement where everyone is comfortable acknowledging when change is necessary. The ability to acknowledge when issues like flawed data collection methods or inconsistent data monitoring arise is monumental for business growth. Recognizing these issues and developing an appropriate response helps preserve your investments and will ultimately show greater returns than a business that is resistant to change.
How to improve data quality for your business
Implementation of data quality technology solutions across a company can streamline and unify data improvement efforts. This will result in the enforcement of consistent data standards across the organization, which means your data can be more easily used for advanced, comprehensive decision-making.
To learn more about how to improve your data quality, download our paper on creating a strong and calculated data quality strategy. We also encourage you to try our email verification and data validation tools to strengthen your dataset. Contact Experian today to see how our tools and services can give you the help you need to collect, maintain and analyze accurate and meaningful data.